$3.83 Billion MLOps Market Boom in 2025: U.S., India, and Germany Lead the AI and Machine Learning Revolution
The MLOps Market is projected to reach $3.83 billion by 2025, driven by AI innovation in the U.S., India, and Germany. Discover key growth factors and industry trends shaping MLOps adoption.
- Last Updated:
MLOps Market Q1 and Q2 2025 Forecast
The MLOps (Machine Learning Operations) market is expected to reach $3.83 billion in 2025, growing at a CAGR of 44.8% from 2025 to 2033. In Q1 2025, the market is projected to generate approximately $915 million, driven by increasing adoption of AI and machine learning automation in the U.S., India, and Germany. Companies are investing in scalable ML deployment, AI-driven analytics, and automated model retraining to enhance decision-making and operational efficiency.
By Q2 2025, the market is expected to reach $960 million, supported by rising demand for cloud-based MLOps platforms, AI governance frameworks, and DevOps integration. The U.S. leads in enterprise AI adoption, while India is witnessing rapid growth due to its expanding AI startup ecosystem. Germany, with its strong industrial base, is leveraging MLOps for manufacturing optimization and predictive maintenance.
As AI models become more complex, enterprises are prioritizing scalable, compliant, and efficient ML lifecycle management through automated pipelines, model monitoring tools, and AI ethics regulations, ensuring robust growth in the MLOps sector.
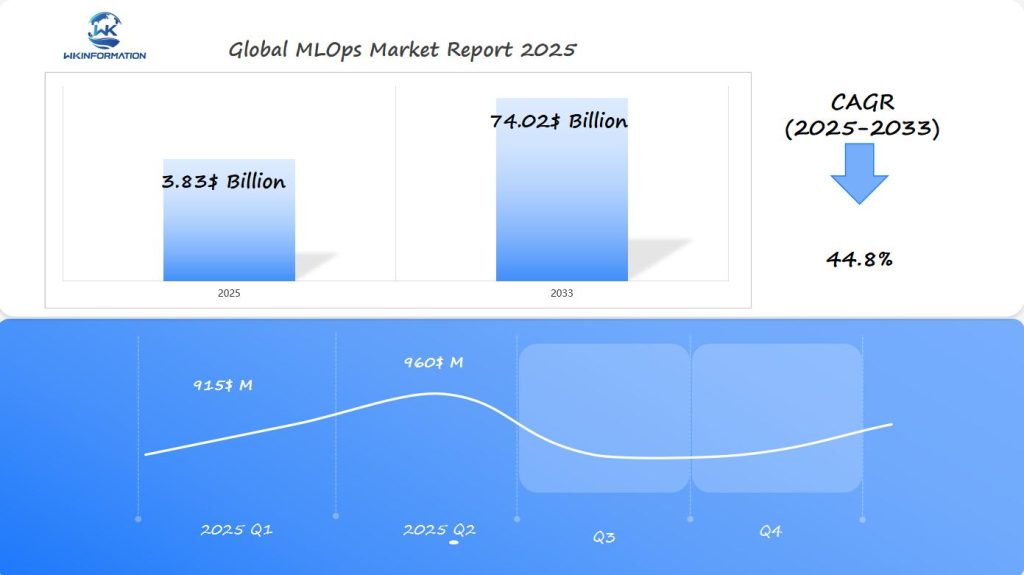
Key Takeaways
- The MLOps market is expected to reach $3.83 billion by 2025.
- The United States, India, and Germany are leading global AI innovation.
- Machine learning operations are transforming enterprise technology strategies.
- Technological advancements are driving market expansion.
- There is an increased focus on streamlining AI deployment processes.
Exploring the Upstream and Downstream Industry Chains for MLOps
The MLOps ecosystem is key in linking machine learning development to its use in operations. It changes how companies handle their machine learning by using advanced DevOps for AI methods.
Important parts of the MLOps ecosystem include several key stages:
- Data preparation and management
- Model development and training
- Model deployment and monitoring
- Continuous integration and delivery
Interconnected Workflow Dynamics
The machine learning lifecycle needs teamwork between data scientists, IT teams, and software engineers. MLOps makes this teamwork easier by setting up standard processes. This leads to better efficiency and growth.
Companies using strong MLOps strategies see big benefits like:
- AI solutions hitting the market faster
- Better model performance and dependability
- Easier governance and following rules
- Better use of resources
Strategic Implementation Approach
Getting MLOps right means taking a complete view that breaks down old barriers between teams. By using teamwork technologies and common frameworks, companies can really make their machine learning work pay off.
“MLOps is not just a technology, it’s a strategic approach to AI development and deployment.” – AI Industry Expert
Key Trends Driving the MLOps Market: Automation, AI, and Continuous Integration
The MLOps world is changing fast thanks to new tech. Companies are using AutoML and AI to make machine learning work better and faster.
- Advanced AutoML platforms simplifying complex model development processes
- AI-driven operations enabling intelligent decision-making
- Robust CI/CD for machine learning accelerating deployment cycles
Automation: Transforming Machine Learning Workflows
AutoML is making machine learning easier for everyone. It automatically selects the most suitable algorithms and configurations, empowering data scientists to tackle complex challenges.
AI-Driven Operational Strategies
Companies are using AI to predict better and stay strong. Machine learning models adjust to new situations. They give insights and make decisions on their own.
Continuous Integration and Deployment Innovations
CI/CD for machine learning keeps models working well. Teams can control versions, test, and deploy quickly.
MLOps Trend | Key Impact | Market Potential |
AutoML | Simplified Model Development | High |
AI-Driven Operations | Intelligent Decision Making | Very High |
CI/CD for ML | Enhanced Deployment Efficiency | Moderate to High |
New tech is making the MLOps market grow and get better.
Challenges in MLOps Implementation and Scalability in Enterprises
Enterprises face significant obstacles in implementing machine learning operations (MLOps) into their systems. They must address complex technical and organizational challenges, which require innovative solutions.
The main MLOps challenges stem from issues related to technology integration and data management. Companies often find it difficult to deploy machine learning models effectively and ensure their continuous optimal performance.
Core Challenges in Enterprise MLOps Implementation
- Data governance complexity
- Model versioning and reproducibility
- Scalability infrastructure limitations
- Compliance and regulatory requirements
Key Scalability Barriers
For enterprise AI to grow, strong infrastructure and smart data management are key. Companies must tackle several big challenges:
- Keeping model performance steady across different settings
- Handling big data quality and integrity
- Creating clear AI governance frameworks
Challenge Category | Primary Impact | Potential Solution |
Data Governance | Model Reliability | Centralized Data Management Platform |
Model Versioning | Reproducibility | Advanced Tracking Systems |
Compliance | Regulatory Alignment | Automated Compliance Checks |
Companies are seeing that MLOps success needs a complete strategy. Investing in infrastructure, talent, and governance is key to beating AI scalability hurdles.
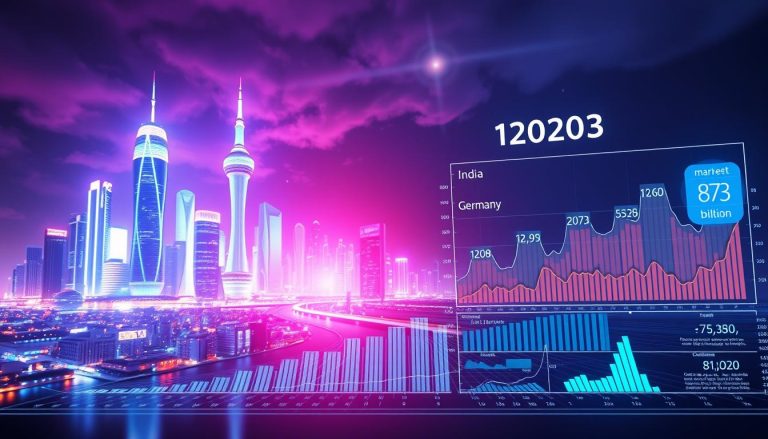
Geopolitical Influence on the MLOps Market
The global MLOps scene is changing fast because of complex politics. These politics shape AI rules and how technology moves across borders. Countries now see machine learning as a key tech area.
- Regulatory framework variations between countries
- International collaboration and competition in AI innovation
- Trade policies affecting technology transfer
- Strategic investments in machine learning infrastructure
Emerging Regulatory Challenges
Different places have their own ways of handling AI rules. The U.S. focuses on letting the market lead, while the EU puts a big emphasis on protecting data and making AI ethical. These different methods make it hard to set up MLOps worldwide.
“The future of MLOps will be defined by how nations balance technological innovation with regulatory oversight.” – AI Policy Research Institute
As AI rules get more complex, moving technology around becomes harder. Big companies have to deal with these tricky laws to stay ahead in the MLOps world.
Region | MLOps Regulatory Approach | Innovation Index |
United States | Flexible, Industry-Led | High |
European Union | Strict, Ethical Framework | Medium-High |
China | State-Controlled | High |
The mix of politics and MLOps is changing the world of technology. It’s pushing innovation but also bringing big challenges in making rules.
MLOps Market by Type: Cloud-Based, On-Premise, and Hybrid Solutions
The MLOps market is growing fast, offering different ways for companies to use machine learning. Cloud MLOps is changing the game for businesses looking for scalable and flexible solutions.
- Cloud-Based MLOps: It’s all about scalability and saving money.
- On-Premise AI Infrastructure: It gives you full control over your data and keeps it safe.
- Hybrid ML Solutions: It’s a mix of flexibility and precision.
Cloud MLOps: Driving Technological Innovation
Cloud MLOps allows businesses to access large amounts of computing power without significant upfront costs. It enables data scientists to work more efficiently and deploy models without being concerned about the underlying technology.
On-Premise AI Infrastructure: Critical for Sensitive Environments
Healthcare and finance require excellent data protection. On-premise AI provides them with the necessary control, making it essential for these industries.
Hybrid ML Solutions: The Best of Both Worlds
Hybrid solutions are the most advanced. They mix cloud benefits with on-premise security. This way, companies can tailor their machine learning setups to fit their needs perfectly.
Applications of MLOps in Healthcare, Finance, and Retail Industries
Machine Learning Operations (MLOps) is transforming major industries with intelligent solutions. It introduces fresh perspectives and improved efficiency to healthcare, finance, and retail sectors. These advancements are primarily driven by cutting-edge AI technologies.
Healthcare AI: Transforming Patient Care
Healthcare AI, powered by MLOps, is making significant advancements in medical care. It utilizes intelligent algorithms for:
- Accurate diagnoses through advanced medical imaging analysis
- Customized treatment recommendations
- Proactive healthcare monitoring
- Efficient management of hospital operations
Financial Machine Learning: Risk Management Innovations
Financial institutions use machine learning to better manage risks and detect fraud. MLOps offers advanced tools for:
- Real-time transaction monitoring
- Automated credit risk evaluation
- Algorithmic trading strategies
- Comprehensive financial fraud prevention
Retail Personalization: Transforming Customer Experiences
Retail personalization with MLOps helps businesses tailor experiences for customers. It includes:
- Intelligent product recommendations
- Dynamic pricing strategies
- Inventory optimization
- Customer behavior prediction
These MLOps applications show AI’s power in different fields. They improve efficiency, personalization, and decision-making.
Global Insights into the MLOps Market
The global MLOps market is changing fast, changing how companies use machine learning. As new AI markets grow, how we use AI is shaped by culture.
Looking at the global MLOps market, we see a few key points:
- How technology is used varies by region
- Culture affects how AI is integrated
- New markets are opening up in machine learning
Regional Innovation Landscape
Every region has its own way of using MLOps:
- North America is all about quick innovation.
- Europe focuses on following rules.
- Asia, especially India and China, is all about fast, affordable tech.
“MLOps is not just a technological solution, but a cultural transformation that varies across global markets.” – AI Research Institute
Cultural Factors Influencing MLOps Adoption
Culture significantly impacts the usage of MLOps. Several factors come into play, including:
- Technological readiness
- Skill levels
- Openness to new ideas within a company
Countries like the US and Germany have robust technological foundations. However, regions such as Southeast Asia and Latin America are quick to adapt to new technologies.
Strategic Market Perspectives
Companies looking to use MLOps globally need to think about:
- The local tech setup
- How skilled the workforce is
- The rules they have to follow
- How much they invest in AI research
The future of MLOps is about understanding and working together across the world. This will make our tech world more connected and innovative.
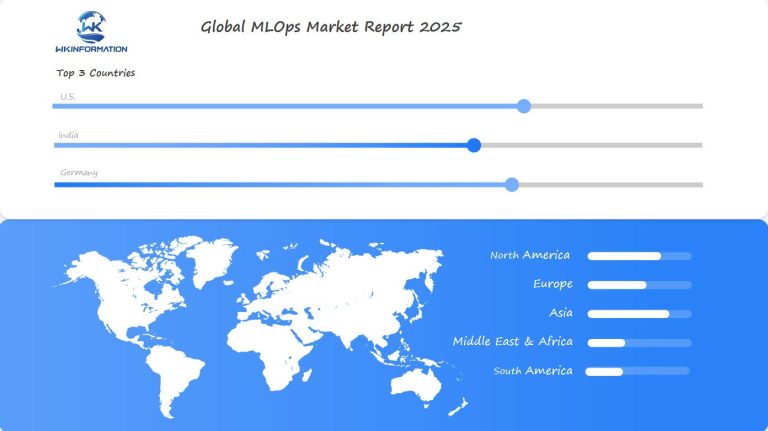
U.S. Pioneering AI and Machine Learning Operations in Diverse Industries
The United States is at the forefront of AI innovation, primarily due to Silicon Valley’s MLOps (Machine Learning Operations). This has resulted in significant transformations across multiple sectors. The U.S. AI leadership plays a crucial role in advancing global technology, revolutionizing industries through the implementation of cutting-edge machine learning techniques.
Several key factors contribute to the United States’ position as a leader in AI and machine learning:
- Strong government support for AI research and development initiatives.
- Substantial investments in AI startups by venture capital firms.
- Presence of top-tier research institutions and thriving tech ecosystems.
Innovation Across Critical Sectors
The AI chip manufacturing landscape shows U.S. tech strength. Companies like NVIDIA, AMD, and new players are making big strides in AI hardware and software.
Industry | MLOps Innovation | Key Players |
Healthcare | Predictive diagnostics | Google Health, IBM Watson |
Finance | Risk assessment algorithms | JPMorgan Chase, Goldman Sachs |
Technology | Advanced machine learning platforms | Microsoft Azure, Amazon Web Services |
Future of Machine Learning Operations
Government AI efforts are speeding up innovation with strategic investments and partnerships. Silicon Valley remains the global center for machine learning research and development. It draws the best talent and leads in new tech breakthroughs.
India’s Growing Role in MLOps for IT and Data Science Innovation
The Indian tech scene is changing fast. Indian AI innovation is becoming a big player in machine learning operations (MLOps). Bangalore, the tech hub, is leading this change, drawing in top talent and investments from around the world.
India’s talent in MLOps is changing the tech world. Indian experts are solving big machine learning problems. They create solutions that meet complex needs in various industries.
Key Drivers of India’s MLOps Ecosystem
- Extensive pool of skilled technology professionals
- Robust startup ecosystem supporting AI innovation
- Significant government investments in digital infrastructure
- Growing venture capital interest in AI technologies
“India is not just participating in the AI revolution—it’s actively shaping the future of technology innovation.” – Tech Industry Expert
MLOps Innovation Area | Key Strengths | Global Impact |
Cloud-based Solutions | Cost-effective Development | Scalable Enterprise Tools |
AI Infrastructure | Advanced Talent Pool | Cutting-edge Research |
Machine Learning Platforms | Rapid Prototype Development | Innovative Problem-solving |
India’s mix of technical skills, entrepreneurial spirit, and investments makes it key in MLOps. Indian technology professionals are not just adapting to change—they are driving it.
Germany’s Advancements in Industrial AI and Machine Learning Systems
Germany is leading in German industrial AI innovation. It’s changing manufacturing and technology. The country is a global leader in smart manufacturing thanks to its focus on Industry 4.0 MLOps.
German AI research is making big strides:
- Advanced robotics in manufacturing
- Smart machine learning for predictive maintenance
- Leading automation for industries
Ethical AI Development in Industrial Contexts
German research focuses on ethical AI development. It’s all about responsible tech use. Universities and tech firms work together to make AI that’s transparent, accountable, and people-focused.
The country’s tech scene follows important rules:
- Strong data privacy laws
- AI governance frameworks
- Watching how algorithms make decisions
Companies like Siemens and Bosch show how AI can improve manufacturing. They use machine learning to make processes better while keeping ethics high.
The Future of MLOps: Hyperautomation, AI Governance, and Real-Time Data Analytics
The world of machine learning operations is changing fast. New technologies are coming along that will change how we use artificial intelligence and data analytics.
Hyperautomation in MLOps is becoming key for companies wanting to make machine learning easier. It uses advanced tech to automate complex tasks with great speed and accuracy.
Key Technological Drivers
- AI governance evolution enabling more transparent and responsible machine learning deployments
- Real-time ML analytics powering instant decision-making capabilities
- Edge computing integration for faster data processing
AI governance is getting better, leading to more ethical and clear machine learning. Companies are focusing on using AI in a way that’s fair and accountable. This helps avoid problems caused by biased algorithms.
Emerging Technological Innovations
Technology | Impact on MLOps | Adoption Rate |
Hyperautomation Platforms | Enhanced workflow automation | High |
Real-Time Analytics Engines | Instant data processing | Medium-High |
AI Governance Tools | Ethical AI deployment | Growing |
Real-time ML analytics are changing how we use data. They give us quick insights in many fields. The use of 5G and edge computing is making these systems even smarter and faster.
Strategic Implications
Companies that invest in hyperautomation and strong AI governance will have big advantages. The future of MLOps is about making systems that can adapt quickly to new situations.
Competitive Landscape in the MLOps Market
The MLOps market is a fast-paced arena where new AI startups and big tech companies fight for the top spot. This scene is filled with quick innovation, smart partnerships, and a push to improve machine learning operations.
Leading players in the MLOps market stand out by tackling tough machine learning problems in unique ways. The AI startup world is booming, with new companies shaking up traditional machine learning methods.
Major Players in the MLOps Market
Here are some of the major players in the MLOps market:
-
IBM – United States
-
Microsoft – United States
-
Google – United States
-
AWS (Amazon Web Services) – United States
-
HPE (Hewlett Packard Enterprise) – United States
-
DataRobot – United States
-
Cloudera – United States
-
Alteryx – United States
-
Domino Data Lab – United States
-
H2O.ai – United States
Overall
Report Metric | Details |
---|---|
Report Name | Global MLOps Market Report |
Base Year | 2024 |
Segment by Type | · On-premises Deployment
· Cloud Deployment |
Segment by Application | · BFSI
· Healthcare · Retail · IT · Energy · Others |
Geographies Covered | · North America (United States, Canada)
· Europe (Germany, France, UK, Italy, Russia) · Asia-Pacific (China, Japan, South Korea, Taiwan) · Southeast Asia (India) · Latin America (Mexico, Brazil) |
Forecast units | USD million in value |
Report coverage | Revenue and volume forecast, company share, competitive landscape, growth factors and trends |
The MLOps market is set for big growth, with predictions of significant expansion in global tech sectors. Investors and companies are seeing the value of machine learning operations in digital transformation. The AI investment trends show a strong ecosystem forming around machine learning.
Recommendations for Companies
Experts recommend investing in cloud-based MLOps solutions. Companies should look for platforms that offer scalable infrastructure and advanced analytics. These platforms should also integrate well with current tech systems. The MLOps market forecast sees big opportunities in healthcare, finance, and manufacturing, where data-driven decisions are key.
Strategies for Technology Vendors
Technology vendors can make the most of this trend by creating adaptable MLOps tools for complex tasks.
Guidance for Investors
Investors should look for companies with strong machine learning governance and innovative automation. These companies should also have a proven track record in AI solutions across various industries.
Investment Opportunities in Emerging Markets
Emerging markets like India and Germany offer exciting investment opportunities for MLOps technologies. Companies that match their tech capabilities with market demands will likely see rapid growth. They will gain a competitive edge in the fast-changing digital tech world.
Global MLOps Market Report (Can Read by Free sample) – Table of Contents
Chapter 1: MLOps Market Analysis Overview
- Competitive Forces Analysis (Porter’s Five Forces)
- Strategic Growth Assessment (Ansoff Matrix)
- Industry Value Chain Insights
- Regional Trends and Key Market Drivers
- MLOps Market Segmentation Overview
Chapter 2: Competitive Landscape
- Global MLOpsplayers and Regional Insights
- Key Players and Market Share Analysis
- Sales Trends of Leading Companies
- Year-on-Year Performance Insights
- Competitive Strategies and Market Positioning
- Key Differentiators and Strategic Moves
Chapter 3: MLOps Market Segmentation Analysis
- Key Data and Visual Insights
- Trends, Growth Rates, and Drivers
- Segment Dynamics and Insights
- Detailed Market Analysis by Segment
Chapter 4: Regional Market Performance
- Consumer Trends by Region
- Historical Data and Growth Forecasts
- Regional Growth Factors
- Economic, Demographic, and Technological Impacts
- Challenges and Opportunities in Key Regions
- Regional Trends and Market Shifts
- Key Cities and High-Demand Areas
Chapter 5: MLOps Emerging and Untapped Markets
- Growth Potential in Secondary Regions
- Trends, Challenges, and Opportunities
Chapter 6: Product and Application Segmentation
- Product Types and Innovation Trends
- Application-Based Market Insights
Chapter 7: MLOps Consumer Insights
- Demographics and Buying Behaviors
- TargetAudience Profiles
Chapter 8: Key Findings and Recommendations
- Summary of MLOps Market Insights
- Actionable Recommendations for Stakeholders
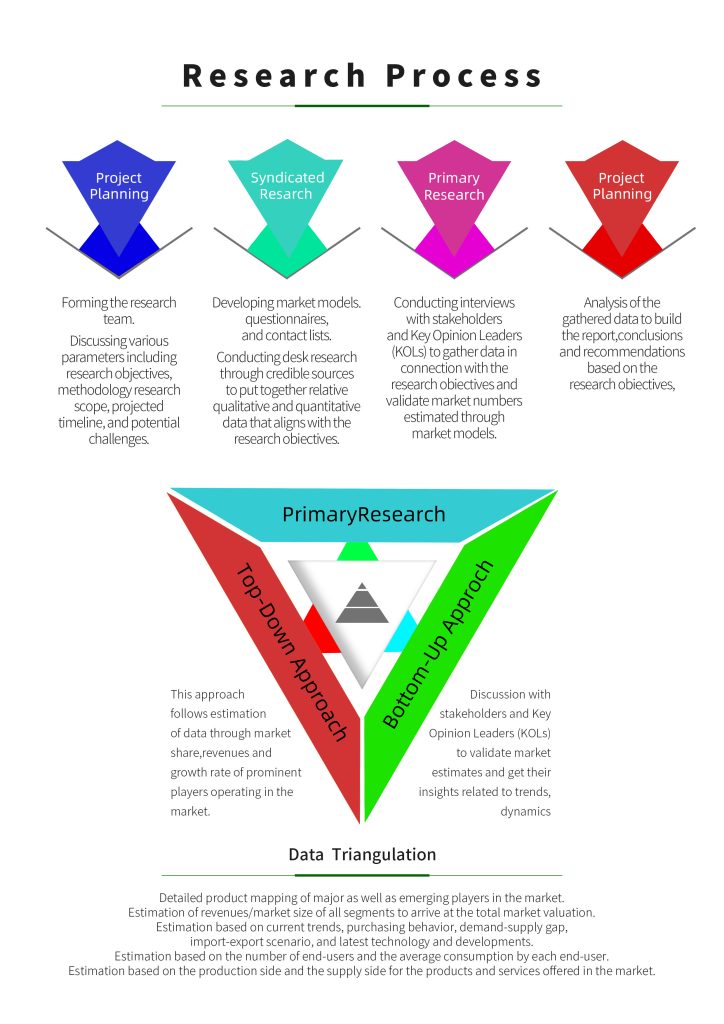
Access the study in MULTIPLEFORMATS
Didn’t find what you’re looking for?
TALK TO OUR ANALYST TEAM
Need something within your budget?
NO WORRIES! WE GOT YOU COVERED!
Call us on: +1-866-739-3133
Email: infor@wkinformation.com
What is the projected market value for MLOps by 2025?
The MLOps market is expected to reach $3.83 billion by 2025, indicating significant growth in the AI and machine learning industry.
Which countries are leading the MLOps innovation?
The United States, India, and Germany are leading in AI and machine learning. They bring unique strengths to MLOps through tech advancements and strategic plans.
What are the key components of the MLOps ecosystem?
The MLOps ecosystem includes data preparation, model development, deployment, and monitoring. These components work together to streamline the machine learning process.
How does MLOps bridge the gap between data scientists and IT operations?
MLOps connects data scientists and IT teams by setting up standard processes. This makes communication better and ensures models work well everywhere.
What is AutoML and its role in MLOps?
Automated Machine Learning (AutoML) makes model development easier. It automates tasks like feature selection and algorithm choice, making AI more accessible and efficient.
What challenges do enterprises face in implementing MLOps?
Companies face issues like managing data quality, model versions, and ensuring AI is ethical. They need smart strategies to overcome these challenges.
How are different deployment types impacting MLOps?
MLOps solutions are evolving with cloud, on-premise, and hybrid models. Each offers benefits like scalability and security, addressing various technical requirements.
Which industries are benefiting most from MLOps?
Industries such as healthcare, finance, and retail are experiencing significant transformations with the implementation of MLOps. AI is playing a crucial role in enhancing various aspects such as:
- Healthcare: Improving diagnostics and treatment plans.
- Finance: Streamlining risk management processes.
- Retail: Enhancing customer experiences through personalized recommendations.
What are the emerging trends in MLOps?
New trends include:
- Hyperautomation
- Advanced AI governance
- Real-time data processing
Edge computing and 5G are also changing machine learning operations.
How are geopolitical factors influencing MLOps development?
Global politics are affecting MLOps through different rules, international partnerships, and tech policies. This shapes innovation and competition worldwide.